Sekvenční metody Monte Carlo
Sekvenční metody Monte Carlo
diplomová práce (OBHÁJENO)
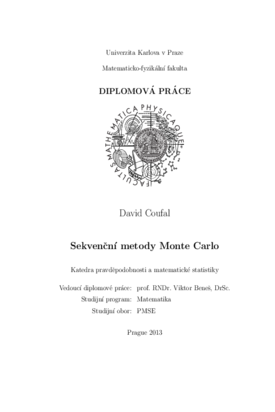
Zobrazit/ otevřít
Trvalý odkaz
http://hdl.handle.net/20.500.11956/55265Identifikátory
SIS: 106507
Kolekce
- Kvalifikační práce [11325]
Autor
Vedoucí práce
Oponent práce
Prokešová, Michaela
Fakulta / součást
Matematicko-fyzikální fakulta
Obor
Pravděpodobnost, matematická statistika a ekonometrie
Katedra / ústav / klinika
Katedra pravděpodobnosti a matematické statistiky
Datum obhajoby
27. 5. 2013
Nakladatel
Univerzita Karlova, Matematicko-fyzikální fakultaJazyk
Angličtina
Známka
Výborně
Klíčová slova (česky)
sekvenční metody Monte Carlo, částicové filtry, neparametrické jádrové odhadyKlíčová slova (anglicky)
sequential Monte Carlo methods, particle filters, nonparametric kernel estimatesNázev práce: Sekvenční metody Monte Carlo Autor: David Coufal Katedra: Katedra pravděpodobnosti a matematické statistiky Vedoucí diplomové práce: prof. RNDr. Viktor Beneš, DrSc. Abstrakt: Práce shrnuje teoretické základy sekvenčních metod Monte Carlo se zaměřením na použití v oblasti částicových filtrů a základní výsledky z oblasti neparametrických jádrových odhadů hustot pravděpodobnostních rozdělení. Přehled výsledků tvoří základ ke zkoumání použití jádrových metod pro aprox- imaci hustot rozdělení částicových filtrů. Hlavními výsledky práce jsou důkaz konvergence jádrových odhadů k příslušným teoretickým hustotám a popis vývoje chyby aproximace v souvislosti s časovou evolucí filtru. Práce je do- plněna experimentální částí demonstrující použití popsaných algoritmů formou simulací ve výpočetním prostředí MATLABR⃝ . Klíčová slova: sekvenční metody Monte Carlo, částicové filtry, neparametrické jádrové odhady
Title: Sequential Monte Carlo Methods Author: David Coufal Department: Department of Probability and Mathematical Statistics Supervisor: prof. RNDr. Viktor Beneš, DrSc. Abstract: The thesis summarizes theoretical foundations of sequential Monte Carlo methods with a focus on the application in the area of particle filters; and basic results from the theory of nonparametric kernel density estimation. The summary creates the basis for investigation of application of kernel meth- ods for approximation of densities of distributions generated by particle filters. The main results of the work are the proof of convergence of kernel estimates to related theoretical densities and the specification of the development of approx- imation error with respect to time evolution of a filter. The work is completed by an experimental part demonstrating the work of presented algorithms by simulations in the MATLABR⃝ computational environment. Keywords: sequential Monte Carlo methods, particle filters, nonparametric kernel estimates