Creating Adversarial Examples in Machine Learning
Vytváření matoucích vzorů ve strojovém učení
diplomová práce (OBHÁJENO)
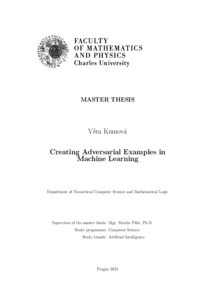
Zobrazit/ otevřít
Trvalý odkaz
http://hdl.handle.net/20.500.11956/124640Identifikátory
SIS: 222687
Kolekce
- Kvalifikační práce [11325]
Autor
Vedoucí práce
Oponent práce
Neruda, Roman
Fakulta / součást
Matematicko-fyzikální fakulta
Obor
Umělá inteligence
Katedra / ústav / klinika
Katedra teoretické informatiky a matematické logiky
Datum obhajoby
4. 2. 2021
Nakladatel
Univerzita Karlova, Matematicko-fyzikální fakultaJazyk
Angličtina
Známka
Výborně
Klíčová slova (česky)
Nepřátelské vzory|Hluboké učení|Klasifikace obrazu|Evoluční algoritmyKlíčová slova (anglicky)
Adversarial examples|{Deep Learning}|{Image classification}|{Evolutionary AlgorithmsTato práce zkoumá nepřátelské vzory ve strojovém učení, konkrétně v oboru klasifikace obrazu. Nejmodernější modely hlubokého učení dokáží rozpoznat vzory lépe než člověk. Nicméně pokud k obrazům přidáme vhodně zvolený šum, můžeme výrazně snížit přesnost daných modelů. Tato práce zkoumá různé metody útoků i obran proti nepřátelským vzorům. Jednu ze současným obran jsme si vybrali, abychom navrhli útok, který ji porazí. Náš útok využívá evoluční algoritmy. Výsledky našich experimentů ukazují, že nepřátelské vzory vytvořené evolucí a vzory vytvořené se znalostí gradientu se výrazně liší. V neposlední řadě zkoumáme, jak jsou naše nepřátelské vstupy přenositelné mezi různými neuronovými sítěmi. 1
This thesis examines adversarial examples in machine learning, specifically in the im- age classification domain. State-of-the-art deep learning models are able to recognize patterns better than humans. However, we can significantly reduce the model's accu- racy by adding imperceptible, yet intentionally harmful noise. This work investigates various methods of creating adversarial images as well as techniques that aim to defend deep learning models against these malicious inputs. We choose one of the contemporary defenses and design an attack that utilizes evolutionary algorithms to deceive it. Our experiments show an interesting difference between adversarial images created by evolu- tion and images created with the knowledge of gradients. Last but not least, we test the transferability of our created samples between various deep learning models. 1