Improvements to Syntax-based Machine Translation using Ensemble Dependency Parsers
Využití kombinace závislostních syntaktických analyzátorů pro zlepšení kvality strojového překladu
dizertační práce (OBHÁJENO)
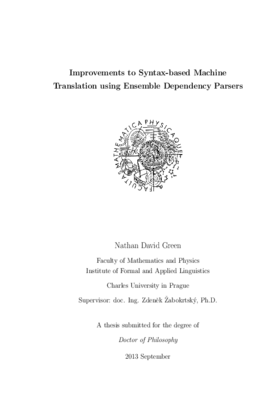
Zobrazit/ otevřít
Trvalý odkaz
http://hdl.handle.net/20.500.11956/57450Identifikátory
SIS: 99980
Kolekce
- Kvalifikační práce [10923]
Autor
Vedoucí práce
Oponent práce
Bick, Eckhard
Zeman, Daniel
Fakulta / součást
Matematicko-fyzikální fakulta
Obor
Matematická lingvistika
Katedra / ústav / klinika
Ústav formální a aplikované lingvistiky
Datum obhajoby
24. 9. 2013
Nakladatel
Univerzita Karlova, Matematicko-fyzikální fakultaJazyk
Angličtina
Známka
Prospěl/a
Závislostní syntaktická analýza věty je jedním za základních výzkumných témat v oblasti zpracování přirozeného jazyka. Výzkum algoritmů pro závislostní analýzu byl dosud zaměřen především na omezené množství jazyků, pro které existuje dostatek trénovacích dat (převážně jde o evropské jazyky). Z dosavadního výzkumu tedy není snadné vyvodit, který algoritmus bude nejvhodnější pro některý další jazyk. Řešení, které navrhujeme, je pracovat místo jednotlivého analyzátoru se souborem několika analyzátorů. Konkrétně zkoumáme tři metody. Zaprvé, sloučíme výstupy jednotlivých analyzátorů do grafu a výsledný závislostní strom sestavíme prostřednictvím hlasování analyzátorů na jednotlivých uzlech. Zadruhé, váhy jednotlivých závislostních hran v grafu určíme na základě seskupení analyzátorů pomocí metody fuzzy clustering, jejímž vstupem je zde rozložení chyb jednotlivých analyzátorů napříč slovními druhy. Zatřetí, implementujeme meta-klasifikátor, který pro každé slovo v dané větě určí nejdůvěryhodnější analyzátor. Závislostní analyzátory, které používáme, jsou založeny na několika odlišných technikách -. jde o grafové analyzátory, přechodové analyzátory a analyzátory spočívající v konverzi ze složkových stromů. Právě tato škála různých analyzátorů nám umožňuje studovat chyby spojené s jednotlivými technikami a vybrat...
Dependency parsing is an integral part of Natural Language Processing (NLP) research for many languages. Research in dependency parsing has mainly dealt with improving accuracy for a limited number of languages. Current de- pendency parsing algorithms have developed mainly for languages with an ample amount of training data. Most of this data has been collected for shared tasks at conferences and are available mainly for European and resource-rich languages. New researchers into the area may not know which algorithm and techniques work best with a new, untested, language. To address this issue, we will look at ensemble approaches to dependency parsing. More specifically, we look at three methods. First, stacking parsers' outputs into a weighted graph and extracting a tree structure using simple voting. Second, analyzing each parsers' errors distribution and using that as an input into the weighted graph through fuzzy clustering methods. Third, using a meta-classifier to choose the best parser for each and every word in our input. The parsers in each situation may come from a variety of techniques such as graph-based, transition-based, and constituent conversion. Using a variety of parsers allows us to study the errors associated with the parsers and choose the best combination or individual parser for each...