Least Absolute Shrinkage and Selection Operator Method
Regresní metoda lasso
bakalářská práce (OBHÁJENO)
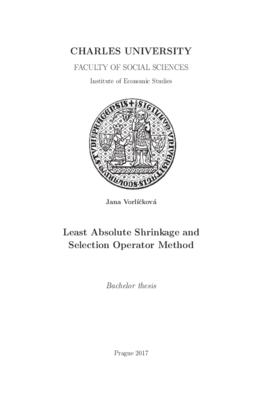
Zobrazit/ otevřít
Trvalý odkaz
http://hdl.handle.net/20.500.11956/85894Identifikátory
SIS: 185493
Kolekce
- Kvalifikační práce [18350]
Autor
Vedoucí práce
Oponent práce
Rusnák, Marek
Fakulta / součást
Fakulta sociálních věd
Obor
Ekonomie a finance
Katedra / ústav / klinika
Institut ekonomických studií
Datum obhajoby
14. 6. 2017
Nakladatel
Univerzita Karlova, Fakulta sociálních vědJazyk
Angličtina
Známka
Výborně
Cílem této práce je diskutovat význam a možné použití penalizačních metod pro lineární model v ekonomii. Penalizace se používá zejména při odhadování parametrů modelu s vysokým počtem proměnných. V této práci se přitom soustředíme na penalizaci nejmenších čtverců, která je nejčastěji používanou ztrátovou funkcí. Penalizační metody zjednodušují model a to tak, že odhady koeficientů u nedůležitých proměnných zmenšují či vynulují, tj. produkují tzv. řídké řešení. Jmenovitě jsou představeny následující metody: hřebenová regrese, úloha výběru nejlepší podmnožiny, lasso metoda a elastická síť. Nejvíce prostoru v teoretické části je věnováno lasso metodě; představeno je i její současné užívání v ekonomických a finančních analýzách. V prak tické části práce analyzujeme reálná ekonomická data pomocí elastické sítě, hřebenové regrese, lasso metody a klasické metody nejmenších čtverců. Získané výsledky srovnáváme pomocí střední čtvercové chyby. Ukazujeme, že pen alizační metody poskytují lepší výsledky než metoda nejmenších čtverců, přičemž nejlepší výsledky jsou získány s pomocí elastické sítě. Klíčová slova penalizované nejmenší čtverce, lasso, elastická síť, hřebenová regrese, pen- alizayní metody v ekonomii 1
The main intention of the thesis is to present several types of penalization techniques and to apply them in economic analyses. We focus on penalized least squares, with a main topic being the lasso. The penalization methods are commonly employed to data sets with a relatively large number of the variables as compared to the sample size. These methods simplify the model by shrinkage of the estimates of the coefficient of the irrelevant variables to wards zero or they put these estimates equal to zero, i.e. they produce a sparse solution. Namely, we present the following methods: ridge regres sion, best subset selection problem, lasso and elastic net. We discuss several applications of the lasso in the current economic and finance research and hence present the lasso in more details. In the practical part of the thesis, we analyze a real economic data using the elastic net, the ridge regression, the lasso and the ordinary least squares method. We use the mean squared error as the measure of performance of the respective method. The penal ized least squares methods surpass the ordinary least squares method, with the elastic net being the best performing method. Keywords penalized least squares, lasso, elastic net, ridge regression, penalization tech niques in economics 1