Aproximativní řešení zobecněných influenčních diagramů
Approximate solution of Unconstrained influence diagrams
diplomová práce (OBHÁJENO)
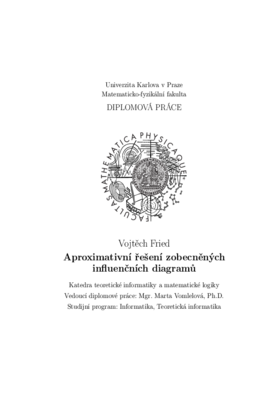
Zobrazit/ otevřít
Trvalý odkaz
http://hdl.handle.net/20.500.11956/7707Identifikátory
SIS: 43292
Kolekce
- Kvalifikační práce [11196]
Autor
Vedoucí práce
Oponent práce
Studený, Milan
Fakulta / součást
Matematicko-fyzikální fakulta
Obor
Teoretická informatika
Katedra / ústav / klinika
Katedra teoretické informatiky a matematické logiky
Datum obhajoby
11. 9. 2006
Nakladatel
Univerzita Karlova, Matematicko-fyzikální fakultaJazyk
Čeština
Známka
Výborně
Podáváme teoretický úvod do problematiky grafických pravděpodobnostních modelů a popisujeme některé jejich typy (bayesovské sítě - BN, Influenční diagramy - ID, zobecněné influenční diagramy - UID). Zobecněné influenční diagramy poskytují možnost volit pořadí rozhodování na základě pozorování. To zvyšuje vyjadřovací sílu UIDu oproti IDum, ale komplikuje to řešení. Přesné řešení UIDu je často nedosažitelné kvůli exponenciální složitosti vzhledem ke klasickému IDu. Navrhujeme a zkoumáme proto některé aproximativní metody řešení UIDu. Výsledkem těchto metod je klasický ID, který vznikne přidáním hran do původního UIDu a měl by mít optimální řešení co nejblíže původnímu UIDu. Jednou ze zkoumaných možností jsou heuristické metody, které vycházejí ze zjednodušeného algoritmu pro hledání optimálního řešení. V průběhu algoritmu fungují jako heuristiky, které umožňují odstranit málo nadějné větve výpočtu. Jinou možností je vytvořit ID přímo. Metody pak experimentálně vyhodnocujeme na náhodně generovaných UIDech tří typů a porovnáváme je zejména s optimálním řešením a se složitostně srovnatelnými metodami založenými jen na náhodě.
We give an introduction to the theory of probabilistic graphical models and describe several types of them (Bayesian Networks - BN, Influence Diagrams - ID, Unconstrained Influence Diagrams - UID). Unconstrained Influence Diagrams support the possibility for the user to choose the ordering of decisions based on observations. This increases the expressive power of UIDs compared to IDs but makes it harder to find an optimal solution. It is often impossible to find an optimal solution because of exponential complexity increase compared to IDs. Therefore we design and investigate several approximate methods to solve UIDs. The result of these methods is an ordinary ID created from the former UID by adding edges. The optimal solution of the ID should be as close to the original UID as possible. Heuristical methods represent one type of the methods investigated. Heuristical methods use a simplification of the optimal algorithm. During the run of the algorithm heuristics are used to cut off the branches that are not perspective for further calculation. Another type of methods is to create the ID directly. We evaluate our methods experimentally based on randomly generated UIDs of three types and compare their performance namely to the optimal solution and to equally complex random methods.