Unsupervised and Semi-Supervised Multilingual Learning for Resource-Poor Languages
Unsupervised and Semi-Supervised Multilingual Learning for Resource-Poor Languages
diplomová práce (OBHÁJENO)
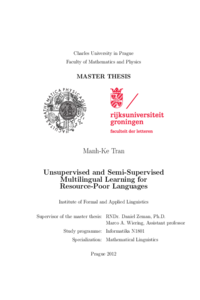
Zobrazit/otevřít
Trvalý odkaz
http://hdl.handle.net/20.500.11956/40830Identifikátory
SIS: 115652
Kolekce
- Kvalifikační práce [11325]
Autor
Vedoucí práce
Konzultant práce
Žabokrtský, Zdeněk
Oponent práce
Vidová Hladká, Barbora
Fakulta / součást
Matematicko-fyzikální fakulta
Obor
Matematická lingvistika
Katedra / ústav / klinika
Ústav formální a aplikované lingvistiky
Datum obhajoby
7. 9. 2012
Nakladatel
Univerzita Karlova, Matematicko-fyzikální fakultaJazyk
Angličtina
Známka
Výborně
Klíčová slova (česky)
přirozený jazyk, strojové učení, morfologie, syntaxeKlíčová slova (anglicky)
natural language, machine learning, morphology, syntaxPra ce se zaměřuje na neř zenou morfologickou segmentaci, jednu ze za kladn ch u loh poč tačov eho zpracov an přirozen eho jazyka. V t eto u loze je c lem rozložit slova na morf emy. Popisuji a reim- plementuji model navrženy v Lee et al. (2011) a vyhodnocuji ho na 4 jazyc ch. Nav c navrhuji generativn model, ktery dok aže využ t reprezentaci slov jako př davn e rysy. Slovn reprezentace jsou rovněž z sk ava ny neř zeny m zp usobem pomoc strojov eho učen a neuronov eho jazykov eho modelu. Pokusy ukazuj , že s využit m těchto př davny ch rys u celkova u spěšnost neř zen eho modelu vzr usta .
This thesis focuses on unsupervised morphological seg- mentation, the fundamental task in NLP which aims to break words into morphemes. I describe and re-implement a model proposed in Lee et al. (2011) and evaluate it on 4 languages. Moreover, I present a generative model that could use word representation as extra fea- tures. The word representations are leant in unsupervised manner using neural language model. The experiment shows that using extra features improves the performance of the unsupervised model.