Machine learning on small datasets with large number of features
Strojové učení na malých datových množinách s velkým počtem atributů
diplomová práce (OBHÁJENO)
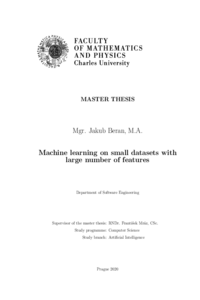
Zobrazit/ otevřít
Trvalý odkaz
http://hdl.handle.net/20.500.11956/119459Identifikátory
SIS: 214664
Kolekce
- Kvalifikační práce [10923]
Autor
Vedoucí práce
Oponent práce
Matzner, Filip
Fakulta / součást
Matematicko-fyzikální fakulta
Obor
Umělá inteligence
Katedra / ústav / klinika
Katedra softwaru a výuky informatiky
Datum obhajoby
8. 7. 2020
Nakladatel
Univerzita Karlova, Matematicko-fyzikální fakultaJazyk
Angličtina
Známka
Výborně
Klíčová slova (česky)
strojové učení, výběr důležitých atributů, klasifikaceKlíčová slova (anglicky)
machine learning, feature selection, classificationPoužitie metód strojového učenia v oblasti biológie je často zložité. Na jednej strane sa v tejto oblasti zvyšuje počet premenných, ktoré je možné zaznamenať. Na strane druhej je však meranie pre každú entitu nákladné, a tak je počet pozorovaní často nízky. V tejto práci sa preto venujeme datasetom, ktoré majú nízky počet pozorovaní, ale veľký počet premenných. Zameriavame sa na rozličné kombinácie metód výberu premenných a klasifikačných metód a pokúšame sa odpovedať na otázku, ktoré kombinácie fungujú najlepšie. Pre lepšie pochopenie súvislostí medzi metódami pracujeme s dvoma simu- lačnými štúdiami a niekoľkými reálnymi datasetmi. Výsledky práce naznačujú, že väčšina klasifikačných metód dosahuje lepšie výsledky, ak pracujú s predvybranými premennými a nie so všetkými premennými dostupnými v dátach. V práci zároveň definujeme hranice pre počet pozorovaní datasetu, od ktorých dosahujú metódy výberu premenných vyššiu kvalitu a stabilitu. V závere práce popisujeme identifikovaný vzťah medzi mierou stabil- ity výberu premenných (tzv. Jaccardov index) a mierou kvality výberu premenných (tzv. False Discovery Rate). 1
Machine learning models are difficult to employ in biology-related research. On the one hand, the availability of features increases as we can obtain gene expressions and other omics information. On the other hand, the number of available observations is still low due to the high costs associated with obtaining the data for a single subject. In this work we, therefore, focus on the set of problems where the number of observations is smaller than the number of features. We analyse different combinations of feature selection and classification models and we study which combinations work the best. To assess these model combinations, we introduce two simulation studies and several real-world datasets. We conclude that most classification models benefit from feature pre-selection using feature selection models. Also, we define model-based thresholds for the number of observations above which we observe increased feature selection stability and quality. Finally, we identify a relation between feature selection False Discovery Rate and stability expressed in terms of the Jaccard index. 1