GPU-accelerated Mahalanobis-average hierarchical clustering
Hierarchické shlukování s Mahalanobis-average metrikou akcelerované na GPU
diplomová práce (OBHÁJENO)
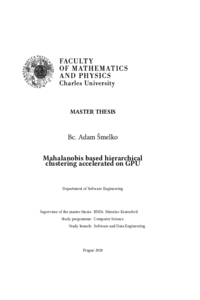
Zobrazit/ otevřít
Trvalý odkaz
http://hdl.handle.net/20.500.11956/119191Identifikátory
SIS: 220193
Kolekce
- Kvalifikační práce [10698]
Autor
Vedoucí práce
Oponent práce
Hric, Jan
Fakulta / součást
Matematicko-fyzikální fakulta
Obor
Softwarové a datové inženýrství
Katedra / ústav / klinika
Katedra softwarového inženýrství
Datum obhajoby
1. 7. 2020
Nakladatel
Univerzita Karlova, Matematicko-fyzikální fakultaJazyk
Angličtina
Známka
Velmi dobře
Klíčová slova (česky)
shlukování, vysokodimenzionální data, GPUKlíčová slova (anglicky)
clustering, high-dimensional data, GPUHierarchické klastrovacie algoritmy sú bežnými nástromi na zjednodušenie, skúmanie a analýzu datasetov v mnohých oblastiach výskumu. Pre prietokovú cytometriu bol navrhnutý špecifický variant aglomeračného zhlukovania, ktorý využíva klastrovanie založené na Mahalanobisovskej vzdialenosti, aby sa dosiahli výsledky, ktoré sa pre danú doménu lepšie hodia. Uplatniteľnosť tohto klastrovacieho algoritmu je v súčasnosti obmedzená jeho pomerne vysokou výpočtovou náročnosťou, ktorá mu neumožňuje škálovať bežné cytometrické datasety. Táto práca popisuje špecializovanú, na GPU- zrýchlenú verziu hierarchického klastrovania založeného na Mahalanobisovskej vzdialenosti, ktorá zlepšuje výkon algoritmu o niekoľko rádov, čo mu umožňuje počítať oveľa väčšie datasety. Práca poskytuje prehľad súčasných hierarchických klastrovacích algoritmov a podrobne popisuje implementáciu algoritmu na GPU. Výsledok sa porovnáva s verejne dostupnými vysokorozmernými údajmi z hmotnostnej cytometrie.
Hierarchical clustering algorithms are common tools for simplifying, exploring and analyzing datasets in many areas of research. For flow cytometry, a specific variant of agglomerative clustering has been proposed, that uses cluster linkage based on Mahalanobis distance to produce results better suited for the domain. Applicability of this clustering algorithm is currently limited by its relatively high computational complexity, which does not allow it to scale to common cytometry datasets. This thesis describes a specialized, GPU-accelerated version of the Mahalanobis-average linked hierarchical clustering, which improves the algorithm performance by several orders of magnitude, thus allowing it to scale to much larger datasets. The thesis provides an overview of current hierarchical clustering algorithms, and details the construction of the variant used on GPU. The result is benchmarked on publicly available high-dimensional data from mass cytometry.