Klasifikace 3D objektů pomocí neuronových sítí
3D object classification using neural networks
diplomová práce (OBHÁJENO)
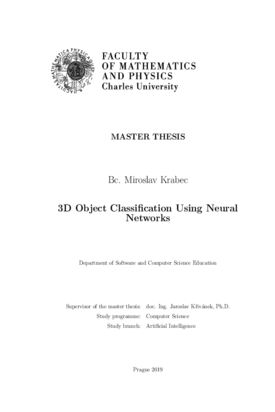
Zobrazit/ otevřít
Trvalý odkaz
http://hdl.handle.net/20.500.11956/108773Identifikátory
SIS: 212509
Kolekce
- Kvalifikační práce [11264]
Autor
Vedoucí práce
Oponent práce
Šikudová, Elena
Fakulta / součást
Matematicko-fyzikální fakulta
Obor
Umělá inteligence
Katedra / ústav / klinika
Katedra softwaru a výuky informatiky
Datum obhajoby
10. 6. 2019
Nakladatel
Univerzita Karlova, Matematicko-fyzikální fakultaJazyk
Čeština
Známka
Velmi dobře
Klíčová slova (česky)
klasifikace objektů, neuronové sítěKlíčová slova (anglicky)
3D objects classification, neural networksKlasifikace 3D objektů pomocí neuronových sítí Bc. Miroslav Krabec Klasifikace 3D objektů se setkává s velkým zájmem v oblasti umělé in- teligence. Existuje mnoho různých přístupů využívajících umělé neuronové sítě k řešení tohoto problému. Liší se hlavně reprezentací 3D modelů, kte- rou neuronové sítě přijímají jako vstup a také svou architekturou. Cílem této práce je prozkoumání a otestování těchto přístupů na veřejně dostupných da- tasetech a jejich podrobení nezávislému srovnání, které se dosud v literatuře neobjevilo. Vytvořili jsme sjednocený framework umožňující převádění dat z běžných 3D formátů. Natrénovali a otestovali jsme deset různých sítí na dvou datasetech (ModelNet40 a ShapeNetCore). Všechny testované sítě podali ro- zumně dobrý výkon, ale většinou nedosáhli přesností (accuracy) uvedených v původních článcích. Domníváme se, že je to způsobeno značným, ale ne- popsaným laděním hyperparametrů na straně autorů původních článků, což naznačuje směr dalšího výzkumu. 1
3D Object Classification Using Neural Networks Bc. Miroslav Krabec Classification of 3D objects is of great interest in the field of artificial intelligence. There are numerous approaches using artificial neural networks to address this problem. They differ mainly in the representation of the 3D model used as input and the network architecture. The goal of this thesis is to explore and test these approaches on publicly available datasets and subject them to independent comparison, which has not so far appeared in the literature. We provide a unified framework allowing to convert the data from common 3D formats. We train and test ten different network on the ModelNet40 and ShapeNetCore datasets. All the networks performed reasonably well in our tests, but we were generally unable to achieve the accuracies reported in the original papers. We suspect this could be due to extensive, albeit unreported, hyperparameter tuning by the authors of the original papers, suggesting this issue would benefit from further research. 1