Transformace stabilizujicí rozptyl
Variance stabilizing transformations
bakalářská práce (OBHÁJENO)
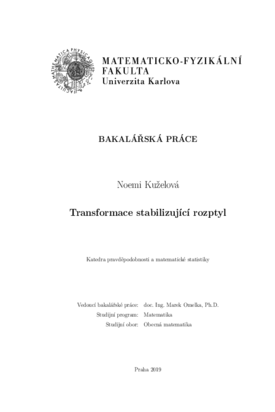
Zobrazit/ otevřít
Trvalý odkaz
http://hdl.handle.net/20.500.11956/108347Identifikátory
SIS: 204367
Kolekce
- Kvalifikační práce [10932]
Autor
Vedoucí práce
Oponent práce
Komárek, Arnošt
Fakulta / součást
Matematicko-fyzikální fakulta
Obor
Obecná matematika
Katedra / ústav / klinika
Katedra pravděpodobnosti a matematické statistiky
Datum obhajoby
27. 6. 2019
Nakladatel
Univerzita Karlova, Matematicko-fyzikální fakultaJazyk
Čeština
Známka
Dobře
Klíčová slova (česky)
transformace stabilizující rozptyl, delta metoda, Poissonovo rozdělení, binomické rozděleníKlíčová slova (anglicky)
variance-stabilizing transformation, delta method, Poisson distribution, binomial distributionAbstrakt. Mnohdy zkoumáme data, jejichž výběrový průměr konverguje k nor- málnímu rozdělení, jehož rozptyl však obecně závisí na neznámém parametru. K tomu, abychom se této závislosti zbavili, lze někdy využít metodu tak zvané transformace stabilizující rozptyl. Tato práce nejprve metodu detailně vysvětlí a najde obecný postup, jak vhodné transformace hledat. Poté se zaměří na data pocházející z Poissonova a binomického rozdělení s neznámými parametry. Pro tato data najde transformace, jež stabilizují (asymptotický) rozptyl, a porovná je s ještě "vylepšenými" transformacemi z článku Anscombe (1948). Právě tvaru těchto transformací je věnována většina práce. Nakonec na simulaci pro výběr z Poissonova rozdělení ukážeme, že je opravdu vhodné tuto metodu využívat a srovnáme odvozenou transformaci s její Anscombeovou verzí.
Abstract. We often examine data whose sample mean converges to a normal distribution, but the variance generally depends on an unknown parameter. To get rid of this dependence, we can sometimes use the so-called variance-stabilizing transformation method. Firstly, this thesis explains the method in detail and finds a general procedure to find suitable transformations. Then it will focus on data from Poisson and binomial distributions with unknown parameters. For these data, it finds transformations that stabilize (asymptotic) variance, and compares them with the "improved"transforms from the article Anscombe (1948). Most of the thesis is devoted to the shape of these transformations. Finally, we show in the Poisson distribution simulation that it is really appropriate to use this method and compare the derived transformation with its Anscombe version.