Klasifikace na množinách bodů v 3D
Klasifikace na množinách bodů v 3D
diplomová práce (OBHÁJENO)
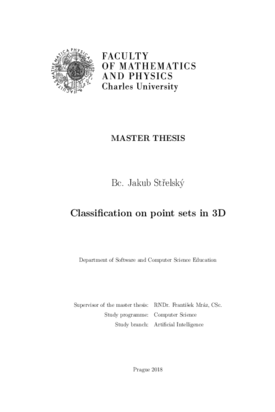
Zobrazit/ otevřít
Trvalý odkaz
http://hdl.handle.net/20.500.11956/101923Identifikátory
SIS: 200056
Kolekce
- Kvalifikační práce [11325]
Autor
Vedoucí práce
Oponent práce
Šikudová, Elena
Fakulta / součást
Matematicko-fyzikální fakulta
Obor
Umělá inteligence
Katedra / ústav / klinika
Katedra softwaru a výuky informatiky
Datum obhajoby
13. 9. 2018
Nakladatel
Univerzita Karlova, Matematicko-fyzikální fakultaJazyk
Angličtina
Známka
Výborně
Klíčová slova (česky)
hluboké učení, klasifikace, neuronové sítěKlíčová slova (anglicky)
deep learning, classification, neural networksRostoucí zájem o klasifikaci 3D geometrických dat vedl k objevu PointNet, což je neuronová síť schopná přímého zpracování neuspořádaných množin bodů. Tato práce prozkoumává několik metod využítí obvyklých bodových příznaků v PointNet síti a jejich účinek na klasifikaci. Účinnost klasifikace předvedených metod byla experimentálně otestována a porovnána s výchozím modelem Point- Net na čtyřech různých sadách dat. Výsledky naznačují, že některé z uvažovaných bodových příznaků mohou vylepšit účinnost klasifikace na datech ve kterých ne- jsou objekty orientovány kanonicky. Obzvláště známé příznaky spin images za- komponované v PointNet síti byly úspěšné v našich experimentech. Objevili jsme navíc alternativu ke spatial transformer, což je součást PointNet sítě, která má za úkol potlačovat problém s nezarovnanou orientací objektů v datech. Další výsledky experimentů naznačují, že tato alternativa může být schopná konkurovat spatial transformer technice na obtížných datech. 1
Increasing interest for classification of 3D geometrical data has led to discov- ery of PointNet, which is a neural network architecture capable of processing un- ordered point sets. This thesis explores several methods of utilizing conventional point features within PointNet and their impact on classification. Classification performance of the presented methods was experimentally evaluated and com- pared with a baseline PointNet model on four different datasets. The results of the experiments suggest that some of the considered features can improve clas- sification effectiveness of PointNet on difficult datasets with objects that are not aligned into canonical orientation. In particular, the well known spin image rep- resentations can be employed successfully and reliably within PointNet. Further- more, a feature-based alternative to spatial transformer, which is a sub-network of PointNet responsible for aligning misaligned objects into canonical orientation, have been introduced. Additional experiments demonstrate that the alternative might be competitive with spatial transformer on challenging datasets. 1