Persistentní homologie a neuronové sítě
Persistent homology and neural networks
diploma thesis (DEFENDED)
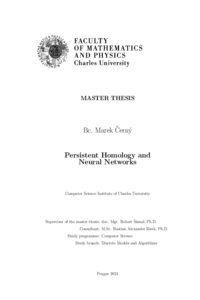
View/ Open
Permanent link
http://hdl.handle.net/20.500.11956/171867Identifiers
Study Information System: 237698
Collections
- Kvalifikační práce [11325]
Author
Advisor
Consultant
Rieck, Bastian Alexander
Referee
Tancer, Martin
Faculty / Institute
Faculty of Mathematics and Physics
Discipline
Discrete Models and Algorithms
Department
Computer Science Institute of Charles University
Date of defense
11. 2. 2022
Publisher
Univerzita Karlova, Matematicko-fyzikální fakultaLanguage
Czech
Grade
Excellent
Keywords (Czech)
homologie|neuronové sítěKeywords (English)
homology|neural networksHluboké učení prakticky vyřešilo nejrůznější problémy počítačového vidění v průběhu poslední dekády. V práci používáme grupy persistentní homologie z výpočetní topolo- gie k analýze prostorů vnitřních representací příznaků v konvolučních neuronových sítích (CNN). Pozorovali jsme souvislost mezi souhrny homologických persistentních grup rozhodovací hranice a chybou tréninku sítě ResNet-18 v průběhu fenoménu hlubokého dvojitého pok- lesu. Navíc jsme se zaměřili na zvlaštní případ souhrnu, který poukazuje na analogii dvojitého sestupu ve směru epoch, což by mohlo vést lepšímu pochopení vnitřních repre- sentací v průběhu kriticky parametrizovaného režimu sítě. V hlavní části navrhujeme a porovnaváme několik přístupů k procesingu samotných modelů CNN sítí pomocí persistentní homologie. Naše nejlepší metoda zvaná Derivo- vatelný persistentní estimátor kvality (DPAE) dosahuje vysoké přesnosti v predikci kval- ity CNN sítí (tj. R2 skore téměř 0.99). DPAE je topologicky optimalizovatelná end-to-end architektura zahrnující derivovatelný výpočet persistence. Co více, DPAE metoda předčí i původní metodu využívající klasického strojového učení, které byla vytvořena přímo pro dataset, který používáme k testování. Veřejně dáváme k dispozici zdrojový kód jak metody DPAE tak ostatních prezentovaných experimentů. 1
Deep learning has solved various computer vision problems in the last decade. We use computational topology, namely persistent homology groups, to analyze the spaces of internal feature representations of convolutional neural networks (CNNs). We observed the correspondence between the summaries of the homological persistence groups of the decision boundary and ResNet-18 CNN training error during the phenomenon of deep double descent. Furthermore, a considered specific persistence summary suggests an analogy to epoch-wise double descent so that we may better understand the internal representations during the critically parametrized regime. Mainly, we develop and compare multiple approaches to CNN models processing using the persistence homology. Our best method, the Differentiable Persistence Accuracy Es- timator (DPAE), achieves very high accuracy in predicting CNN's performance (R2 score close to 0.99). Our DPAE is a topologically optimized end-to-end architecture involving differentiable persistence computation. Moreover, DPAE overperforms the original clas- sical machine learning-based method on its native Small CNN Zoo dataset. We publicly release the complete source code of DPAE and other presented experiments. 1