Deep Neural Networks in Image Processing
Hluboké Neuronové Sítě ve Zpracování Obrazu
diploma thesis (DEFENDED)
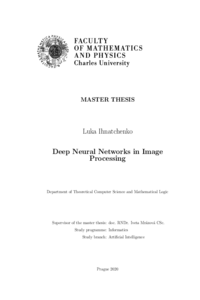
View/ Open
Permanent link
http://hdl.handle.net/20.500.11956/120941Identifiers
Study Information System: 213191
Collections
- Kvalifikační práce [11325]
Author
Advisor
Referee
Pilát, Martin
Faculty / Institute
Faculty of Mathematics and Physics
Discipline
Artificial Intelligence
Department
Department of Theoretical Computer Science and Mathematical Logic
Date of defense
14. 9. 2020
Publisher
Univerzita Karlova, Matematicko-fyzikální fakultaLanguage
English
Grade
Very good
Keywords (Czech)
hluboké neuronové sítě, klasifikace, reprezentace znalostí, CNN-sítě, zpracování obrazuKeywords (English)
deep neural networks, classification, knowledge representation, CNN-networks, image processingCı́lem této diplomové práce bylo navrhnout vhodnou strategii pro detekci a klasifikaci objektu na mamografických obrázcı́ch. Součástı́ tohoto cı́le je im- plementace experimentálnı́ho frameworku, který bude použit pro přı́pravu dat, učenı́ a porovnánı́ modelů. Při analýze byla použita verze datasetu s useknutými a uplnymi obrazky. Inicializace pomocı́ vah, které byly předběžně přetrénované na obrázcı́ch z jiné domény, zlepšily výkon klasifikátoru. ResNet-34 měl lepšı́ AUC skóre na testovacı́ sadě než ResNet-18. Učenı́ s učitelem a bez učitele s využitı́m minimalizace entropie nemá oproti učenı́ pouze s učitelem žádné významné zlepšenı́. Práce obsahuje vizualizaci predikcı́sı́tě a analýzu representaci znalosti klasifikátoru. Dosažené výsledky pro dataset s useknutými obrázky jsou srovnatelné s výsledky jiného článku, který použil stejnou testovacı́sadu. Pro dataset s úplnými obrázky vysledky trénovánı́ byly suboptimálnı́. 1
The goal of this master thesis was to propose a suitable strategy to detect and classify objects of interest in mammogram images. A part of this goal was to implement an experimentation framework, that will be used for data preparation, model training and comparison. Patch and full-image versions of the dataset were used in the analysis. Initialisation with weights that were pretrained on the images from other domain improved classifier performance. ResNet-34 had better AUC scores on the test set that ResNet-18. Semi-supervised training using entropy minimisation has no significant improvement over the supervised one. The thesis includes the visualisation of the network predictions and the analysis of the knowledge representation of the classier. The achieved results for a patch version of the dataset are comparable to the results of another article that utilised the same test set. For a full-image dataset the results of the training were suboptimal. 1