Neural networks and tree-based credit scoring models
Neuronové sítě a stromové metody v kreditních skóringových modelech
bachelor thesis (DEFENDED)
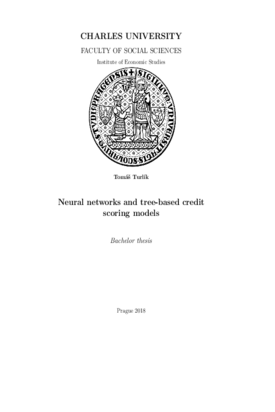
View/ Open
Permanent link
http://hdl.handle.net/20.500.11956/102481Identifiers
Study Information System: 191428
Collections
- Kvalifikační práce [18442]
Author
Advisor
Referee
Fanta, Nicolas
Faculty / Institute
Faculty of Social Sciences
Discipline
Economics and Finance
Department
Institute of Economic Studies
Date of defense
11. 9. 2018
Publisher
Univerzita Karlova, Fakulta sociálních vědLanguage
English
Grade
Excellent
Keywords (English)
machine learning, loan default model, logistic regression, random forests, neural networksJednou z najzákladnejších úloh kreditného skóringu je klasifikácia poten- cionálnych klientov žiadajúcich o úver na "dobrých" alebo "zlých", na zák- lade pravdedepobnosti, že by neboli schopní splácať úver v prípade, že by im bol odsúhlasený. V tejto práci porovnávame často použivanú logistickú regresiu, neuronové siete a ensemble modely založené na stromových metó- dach. Pri konštrukcii neuronových sietí používame nové metódy a poznatky z oblasti hlbokého učenia, zaťiaľčo v prípade stromov používame populárne ensemble algoritmy bagging, boosting a náhodné lesy. Modely porovnávame na základe ROC AUC miery, ktorá by mala poskytnúť väčšiu informačnú hodnotu ako len samotná presnosť. Výsledky naznačujú malý alebo takmer žiadny rozdiel medzi modelmi. V najlepšom prípade, dosahujú neuronové siete, boosted ensemble modely a zložené ensemble modely len približne o 1% − 2% väčšiu ROC AUC hodnotu ako logistická regresia. Klíčová slova kreditní skóring, neuronové sítě, rozhodovací strom, bagging, boosting, náhodný les, ensemble, ROC křivka
The most basic task in credit scoring is to classify potential borrowers as "good" or "bad" based on the probability that they would default in the case they would be accepted. In this thesis we compare widely used lo- gistic regression, neural networks and tree-based ensemble models. During the construction of neural network models we utilize recent techniques and advances in the field of deep learning, while for the tree-based models we use popular bagging, boosting and random forests ensembling algorithms. Performance of the models is measured by ROC AUC metric, which should provide better information value than average accuracy alone. Our results suggest small or even no difference between models, when in the best case scenario neural networks, boosted ensembles and stacked ensembles result in only approximately 1%−2% larger ROC AUC value than logistic regression. Keywords credit scoring, neural networks, decision tree, bagging, boosting, random forest, ensemble, ROC curve